Funded by the 1851 Royal Commission
Summary:
In the UK, poor air pollution stands out as a significant threat to public health, responsible for an estimated 29,000 to 43,000 deaths annually among other harmful health effects. This challenge is underscored by the United Nations’ Sustainable Development Goal 11.6 ‘Reducing the Environmental Impact of Cities’. The introduction of Clean Air Zones (CAZs) across many UK cities aims to improve air quality and public health by discouraging the use of polluting vehicles or other policy interventions. Evaluating the effectiveness of various policies relies on AI-driven insights derived from data acquired through distributed air quality monitoring stations.
However, due to the unique characteristics of urban areas, such as the type and percentage of different emission sources, the intellectual and cognitive capabilities need to be integrated to customise of the Artificial General Intelligence (AGI) solution and support the self-programming. Taking advantage of the impressive capabilities of humanlike understanding and reasoning, two large language models will be trained, namely the reasoning agent and coding agent. Through a conversational interface, the reasoning agent combines the complicated human intent with accumulated knowledge encoded, translated into the Chain-of-Thoughts (CoTs). CoTs, as intermediate reasoning steps, are sequentially delivered to realise the intervention assessment. Finally, the coding agent transforms CoTs into executable codes tailored to the CAZ’s circumstances.
Without the roles of data scientists and programmers, this transition towards self-programming AGI puts stakeholders in the driving seat, improving the transparency and explainability of the AI solution to CAZ stakeholders and the trustworthiness of the solution.
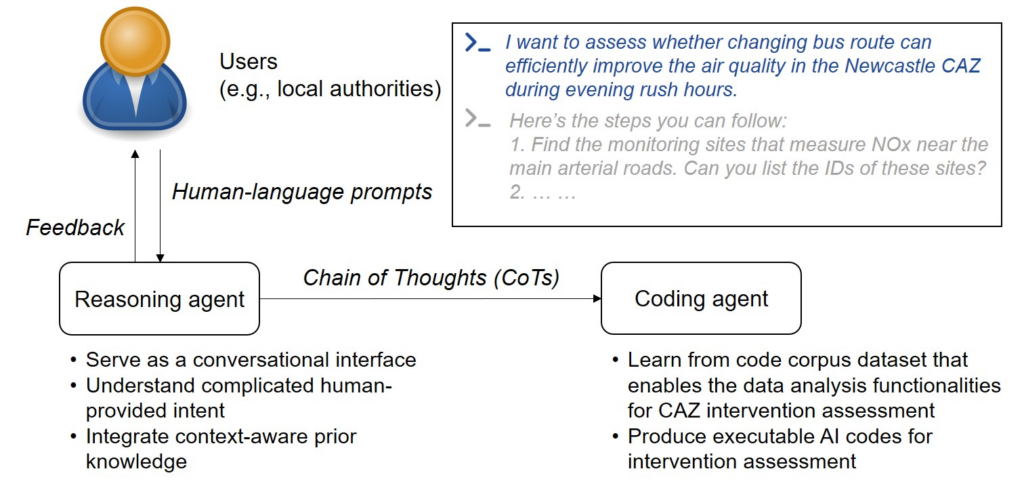